Data analytics-based maintenance solutions for military vehicles
StoryAugust 05, 2021
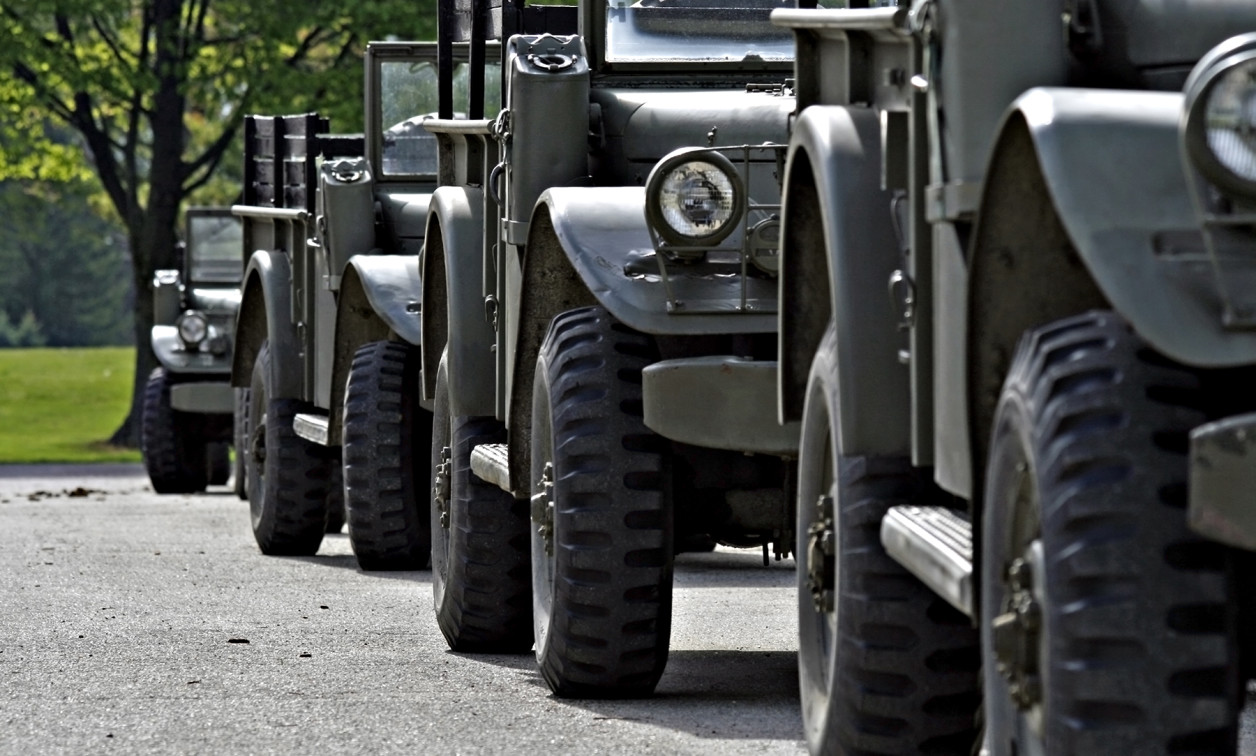
By John D. Price, Lone Star Analysis
Military vehicles are maintained in a variety of circumstances. Whether at a depot or deployed, the goal is operational availability (known as Ao). However, current standards and practices in preventive and corrective maintenance typically occur too early or too late to provide the best Ao within resource constraints. Maintainers have limited insight to each component’s useful life. Because of these timing challenges, current processes can leave shortfalls in Ao and readiness. These shortfalls can be minimized by implementing advanced maintenance analytics.
Using hybrid artificial intelligence (AI), combining machine learning and physics-based AI, maintainers can focus on the state of each vehicle. This is enabled by predictive and prescriptive maintenance software that conducts continuous assessments of equipment health, performs real-time predictive analytics and then prescribes maintenance actions. By leveraging these data tools, organizations can improve mission readiness, reduce equipment failure, and minimize avoidable costs.
Every vehicle-fleet operator struggles to ensure that assets are productive, minimize maintenance and repair costs, and optimize replacement of consumable items such as tires and brakes. When dealing with military vehicles, there is added stress to maintain the operational availability (Ao) of the vehicle fleet. In the case of military logistics, the term “availability” encompasses more than just the ability to be used. Not only does an asset need to be available, but it also needs to be suitable for the specific missions. For example, if a fully operational Humvee is waiting to have armor added so it can be used in hazardous areas, it would be considered unavailable for the specific mission or similar missions. Failure to achieve and maintain a high Ao, due to discrepancies in what’s available as opposed to what is needed, can result in operational mission failure or casualties, neither of which are acceptable.
There are too many interacting variables for a maintainer to effectively monitor all these issues; personnel can only provide limited monitoring of historical data for vehicle fleets. In contrast, computer systems can provide constant evaluation, and with enough machine intelligence, predictions become powerful. Software can deal with the dull, dirty work of sifting through data and vehicle use to prescribe condition-based actions.
Military maintenance faces a dilemma – looming fiscal austerity is being exacerbated by the introduction and acquisition of technologically complex systems. But in an increasingly dangerous world, the requirement to foresee and minimize repairs and maintenance is crucial to both mission accomplishment and resource stewardship.
Important differences exist in the analytics of assets owned by civilians and defense operators: For one, military vehicles tend to be older and less digitally enabled, which affects the amount and types of data available for analysis processes. Military assets also carry security protocols preventing data sharing between systems, if it’s allowed at all. This then creates the dilemma of wanting large amounts of data but not being able to collect or access it.
Civilian fleet-management software – based on original equipment manufacturer (OEM) manuals, abundant data, and operation on good roads – simply can’t cope with the realities of current military vehicles.
Current maintenance practices
Traditional vehicle maintenance follows the path prescribed by the OEM or the military in-service engineering agent (ISEA). In the case of most military equipment, both the OEM and ISEA test the equipment in an attempt to anticipate availability impacts based on projected operating environments. Component service life is established during testing, but these estimates are only averages, and are conversative to avoid component failure which might catastrophically impact the system or personnel operating it. This time-based approach translates into a common practice known as preventive maintenance checks and services (PMCS).
Within this practice, maintenance is carried out at predetermined intervals or according to prescribed criteria, with the goal of reducing the failure risk or performance degradation of the equipment. Maintenance cycles are then planned according to the need to take a device out of service.
While this form of maintenance is beneficial, since its preventive nature reduces chances of unexpected failure, the calendar- or time-based aspect has proven to be unreliable, based on operating environments that may not have been anticipated during acceptance testing. This situation can lead to even more conservative life cycle estimations. When inspected after removal, sometimes components demonstrate a considerable amount of remaining service life. Whether intentional or not, when an organization’s maintenance is dependent on some combination of usage and the calendar, it amounts to choosing to limit an asset’s lifespan and thus allocating too many resources to maintenance. This choice has both operational and cost impacts.
In spite of preventative maintenance, failures do occur, of course, which then require corrective maintenance. Anomalies may be detected during the course of PMCS or may be due to component failure; either case requires action to resolve the issue.
In some cases, operating until failures occur is the maintenance strategy of choice. This method operates on the concept of reducing unnecessary action, as opposed to the preventive nature of PMCS. However, without analytics in place to inform which parts are on the verge of failure, operators run the risk of being unprepared without spare parts or maintenance personnel. In a military setting, this approach also places assets at risk of being stranded in a troublesome area, requiring additional equipment and personnel to recover the asset.
Ultimately, neither strategy truly optimizes the maintenance cycle with one requiring action too soon and the other requiring action too late. A more efficient solution focuses less on the possibility or lack thereof of a component breaking down, and more on how components within each vehicle in a fleet are performing in real time.
Condition-based maintenance
Condition-based maintenance (CBM) represents a significant improvement over both traditional maintenance strategies. CBM can be thought of as an enhancement to preventive maintenance. CBM focuses on replacing components based on their individual conditions rather than mileage or time duration.
CBM delivers an 8% to 12% improvement over normal time or cycle-based preventative maintenance by accurately predicting time to failure. According to the U.S. Department of Energy, it also decreases unforeseen breakdowns by 70% to 75%, and reduces downtime by 35% to 45%.
With a CBM solution, the actual equipment condition is continuously estimated for proper operation and for remaining useful life. When equipment deterioration and the failure probability increases, then maintenance action is taken, with action tailored to the circumstances. A deployed unit might want to be more conservative in avoiding a breakdown than a training unit, for example.
Previously, the technology to perform this type of analytics was unavailable. Older methods were a barrier to more efficient maintenance, especially for military organizations. A remaining barrier in many organizations is the belief that large data pools are needed to perform accurate analysis. The lack of digital data feeds from many military vehicles is at the heart of this mindset. However, physics-based artificial intelligence (AI) solutions only need modest amounts of data or modest upgrades to military vehicles to perform powerful predictive maintenance. Demonstrations have shown powerful CBM analytics using existing (and very limited) data.
Data can drive maintenance actions
AI-driven vehicle condition-based maintenance (VCBM) software monitors every aspect of a vehicle, from air intake to exhaust and all points therein. Leveraging both predictive and prescriptive analytics, VCBM software not only provides continuous diagnostics, but it also delivers problem prediction and solution prescription, enabling personnel to focus on making precise adjustments to optimize the Ao of a military vehicle fleet. Deployable via sensors or vehicle upgrades, a VCBM system performs frequent evaluations on vehicle subsystems such as the internal combustion engine, suspension and chassis, electrical system, fuel system, and lubrication system. It then uses real-time analysis to ensure rapid-onset issues are identified prior to failure.
The analytics performed on each subsystem are based on known physical relationships and equations, either commonly accepted or drawn directly from well-regarded texts and data sources within the automotive industry. By plugging in known information, the software can focus the machine learning on finding unknown information. This approach reduces the need for large data sets and decreases the amount of work necessary for the AI to produce actionable insights.
For example, the internal-combustion engine subsystem has the ability to track the air-fuel ratio and monitor the ranges it operates in while at the same time filtering out operational states, such as the differences seen at low engine speeds and throttle openings versus maximum engine speed and torque demand. (Figure 1.)
[Figure 1 | Marines refuel light armored vehicles during an exercise at Marine Corps Air Ground Combat Center in California. Marine Corps photo by Lance Cpl. Quince Bisard.]
Additionally, when not available from the vehicle’s engine control unit (ECU), the engine load is calculated and tracked over time. Expected brake horsepower and torque are computed, as well as brake-specific fuel consumption and theoretical brake mean effective pressure. Operational observations are also made, such as tracking idle time or time spent at full throttle near the engine’s peak torque output. Engine-specific rules can be applied, such as if the engine has specific shutdown idle time criteria to be met. The mileages of major components are tracked, such as the valve cover gasket, serpentine belt, and other components. Basically, where a condition which advances the degradation of a particular component is known, the degradation rate is applied to the component when the condition is met.
System-failure correlation is also performed where relationships are well-known. For example, a tire with a low-pressure issue will have a higher rolling resistance, increasing the fuel consumption above the normal operating range. Rather than pointing to the engine operating in a degraded state, the root cause can be identified here due to sensor data. In situations where sensors are not available to determine the root cause, the prescription issued for the event will still suggest a manual inspection of other components for functionality to isolate the root cause.
In all systems, a defined health state is applied individually based on the operating characteristics of the specific system. In the instance of the electrical system, health is based on the performance of the alternator and the charge of the battery, whereas in the lubrication system health is based on oil/oil-filter life but can also be affected by oil pressure. If oil pressure drops while the engine is running, the system automatically goes into a critical health state. The overall asset health is an aggregation of all the systems’ health states.
Unlike preventive and corrective maintenance, this approach delivers optimal Ao by monitoring key elements of a vehicle, understanding the relationships between those elements, and enabling consistent vehicle health. In the long term, this data-driven strategy enables organizations to drastically reduce unplanned maintenance impacts, improve safety performance, and maximize fleet utilization, efficiency, and service life.
In a military context, operational availability and mission readiness are complimentary to each other and are crucial elements to mission success. Deploying a reliable and accurate maintenance approach is necessary to ensuring both requirements are met across individual assets as well as entire fleets. Although there are maintenance practices already in place within military organizations to ensure the vehicle availability, new and improved data-centric, AI-driven methods can increase component useful life, reduce probability of failure, and optimize available resources.
John Price has been at Lone Star Analysis since 2018, supporting clients in the transportation and logistics space from solution development and implementation through to deployment and full-scale production. He began his professional career at Lone Star after graduating with a degree in mechanical engineering and a certificate in automotive engineering from the University of Texas at Arlington. He can be contacted at [email protected].
Lone Star Analysis • https://www.lone-star.com/