GUEST BLOG: Deploying AI at the edge: Enhancing military readiness and response
BlogOctober 10, 2024
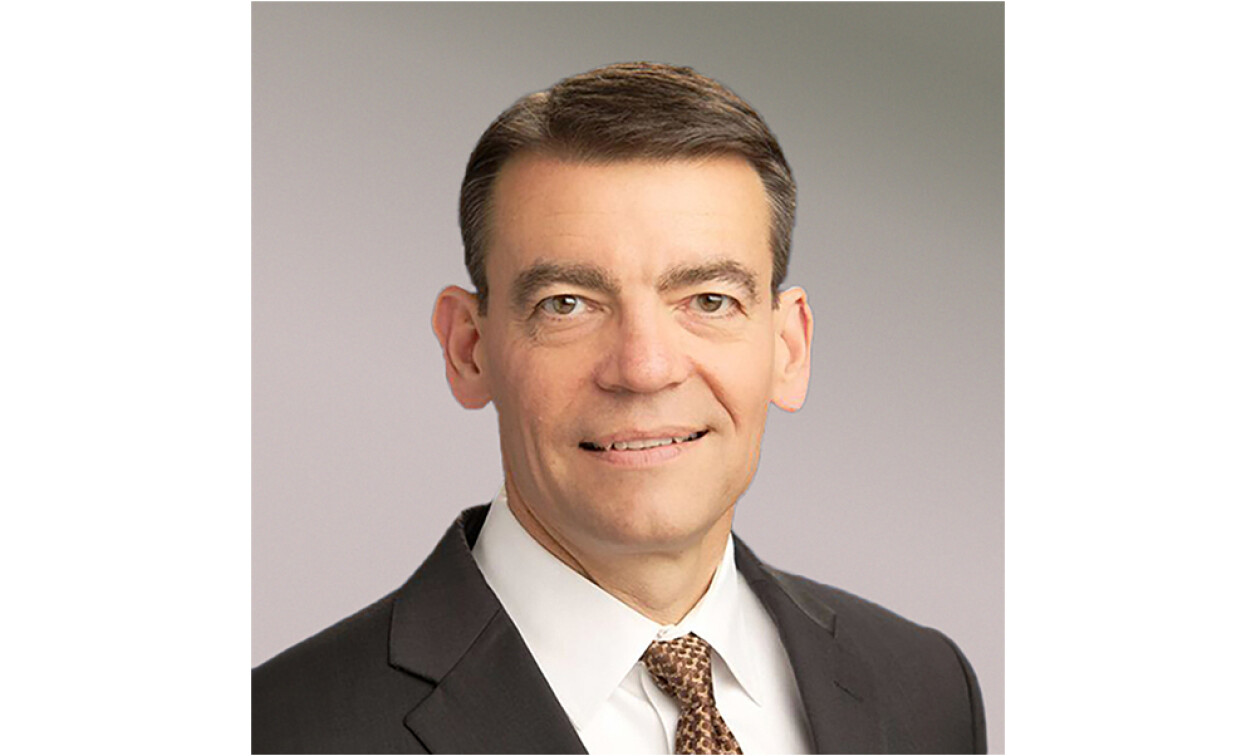
There is a lot of talk about terms like the military edge and artificial intelligence (AI) and their impact on military capability. Many of these conversations start with questions like “What is the edge?” “What is artificial intelligence?” and “What do edge computing and AI imply for embedded systems in the defense market?” Edge computing and AI are two powerful technologies that, when combined, significantly enhance operational capabilities.
Edge computing is the processing of data closer to the source rather than in centralized data centers or cloud environments. An example of edge computing is real-time data analytics on the battlefield, such as soldier-worn sensors processing data locally to make immediate decisions. The term “tactical edge” further emphasizes characteristics of this environment, including right on the front lines or in remote and disconnected locations where time-sensitive computation is needed and communications may be intermittent.
Historically, data collection and analysis have relied heavily on human interpretation. Implementing AI into military systems drastically increases operational capability, delivering faster, more intelligent decisions at the edge. AI also enables more efficient resource use and greater autonomy in constrained edge environments.
An example is the ability for satellites and aircraft to collect more data than they can transmit to the ground for processing. Onboard these platforms, AI image recognition and classification can process imagery immediately and pass along only the most pertinent information to users, reducing latency and required communication bandwidth.
The industry is witnessing increasing trends toward deploying AI at the edge, with the moves now informing major U.S. defense programs. The U.S. Department of Defense (DoD) “Replicator” program and the Air Force’s Collaborative Combat Aircraft program are aiming to create uncrewed wingmen or even fleets that would operate autonomously without constant control by ground-based command centers.
There are several advantages to AI inference at the edge in military operations. Some examples include:
- Advanced autonomous systems: AI-enabled drones or autonomous vehicles capture and process data during complex missions without delays or central processing. This level of autonomy limits the risk to human lives while preserving real-time access to intelligent information.
- Data privacy and improved security: Processing sensitive data locally limits the potential for data breaches during data transmission. Cybersecurity tools can use edge AI to help spot probing patterns before full-scale attacks develop. Once an attack begins, AI close to the point of the cyberattack can guide system and network responses to mitigate damage faster than human personnel can respond.
- Greater reach and operational flexibility: Without the need for constant connectivity to data centers or cloud access, soldiers can process and analyze data even in the most remote locations.
- Improved surveillance: While on the battlefield, personnel can process intelligence, surveillance, and reconnaissance (ISR) data locally with smarter, faster decision-making.
- Real-time data processing and reduced latency: On the battlefield, speed and accuracy is critical when it comes to making lifesaving decisions. Every second counts in these situations: Edge delivers real-time processing without unwanted delays of sending data to and from an off-site location.
However, deploying AI at the edge does bring some challenges, including platform size, weight, and power (SWaP) constraints plus the need for cooling equipment. These issues are further heightened by the increased computational demand in modern generative AI workloads. For these combined technologies to enhance military readiness and response, defense systems need to leverage highly efficient, edge AI coprocessors that can handle both general AI and generative AI workloads at the edge. Such systems enable high-performance compute architectures to meet the demanding workload requirements of current and future battlefields while performing within the operational limits of tactical-edge platforms.
Stanley Crow is Vice President of Defense & Space Technology at EdgeCortix.
EdgeCortix • https://www.edgecortix.com/en/